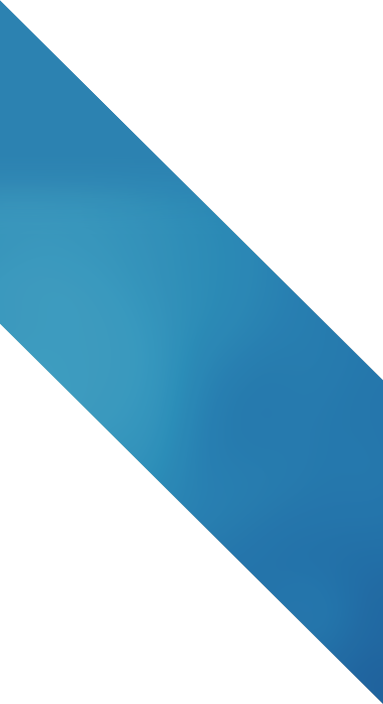
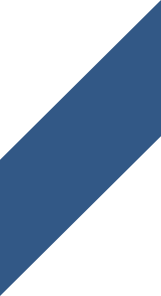
Improvement in Supply Chain Planning Accuracy
1
%
Reduction in Planning Time
1
%
We use cookies on our website to give you the most relevant experience by remembering your preferences and repeat visits. By clicking “Accept All”, you consent to the use of ALL the cookies. However, you may visit "Cookie Settings" to provide a controlled consent.
Necessary cookies are required to enable the basic features of this site, such as providing secure log-in or adjusting your consent preferences. These cookies do not store any personally identifiable data.
Functional cookies help perform certain functionalities like sharing the content of the website on social media platforms, collecting feedback, and other third-party features.
Analytical cookies are used to understand how visitors interact with the website. These cookies help provide information on metrics such as the number of visitors, bounce rate, traffic source, etc.
Performance cookies are used to understand and analyze the key performance indexes of the website which helps in delivering a better user experience for the visitors.
No cookies to display.
Advertisement cookies are used to provide visitors with customized advertisements based on the pages you visited previously and to analyze the effectiveness of the ad campaigns.
Other cookies are those that are being identified and have not been classified into any category as yet.
Leading companies across numerous industries use Gurobi’s mathematical optimization solver – in a wide variety of applications – to optimize their supply chain planning, decision making, and operations and keep supply and demand in balance.
With mathematical optimization, you can:
Gurobi delivers blazing speeds and advanced features—backed by brilliant innovators and expert support.
With our powerful algorithms, you can add complexity to your model to better represent the real world, and still solve your model within the available time.
Our development team includes the brightest minds in decision-intelligence technology--and they're continually raising the bar in terms of solver speed and functionality.
Our PhD-level experts are here when you need them—ready to provide comprehensive guidance and technical support. They bring deep expertise in working with commercial models and are there to assist you throughout the process of implementing and using Gurobi.
Dive deep into sample models, built with our Python API.
In this example, we’ll show you how to solve a goal programming problem that involves allocating the retailers to two divisions of a company in order to optimize the trade-offs of several market sharing goals. You’ll learn how to create a mixed integer linear programming model of the problem using the Gurobi Python API and how to find an optimal solution to the problem using the Gurobi Optimizer. This model is example 13 from the fifth edition of Model Building in Mathematical Programming by H. Paul Williams on pages 267-268 and 322-324. This modeling example is at the beginner level, where we assume that you know Python and that you have some knowledge about building mathematical optimization models. You may also want to check out the documentation of the Gurobi Python API.
Learn MoreIn this example, you’ll learn how to tackle one of the most famous combinatorial optimization problems in existence: the Traveling Salesman Problem (TSP). The goal of the TSP – to find the shortest possible route that visits each city once and returns to the original city – is simple, but solving the problem is a complex and challenging endeavor. We’ll show you how to do it! This modeling example is at the advanced level, where we assume that you know Python and the Gurobi Python API and that you have advanced knowledge of building mathematical optimization models. Typically, the objective function and/or constraints of these examples are complex or require advanced features of the Gurobi Python API.
Learn MoreMathematical optimization uses the power of math to find the best possible solution to a complex, real-life problem. You input the details of your problem—the goals you want to achieve, the limitations you’re facing, and the variables you control—and the mathematical optimization solver will calculate your optimal set of decisions.
80% of the world’s leading companies use mathematical optimization to make optimal business decisions. For example, Air France uses it to build the most efficient schedule for its entire fleet, in order to save on fuel and operational costs, while reducing delay propagation.
Descriptive and predictive analytics show you what has happened in the past, why it happened, and what’s likely to happen next. But to decide what to do with that information, you need human input—which can introduce bias.
With mathematical optimization, you receive a decision recommendation based on your goals, constraints, and variables alone. You can, of course, involve human input when it comes to whether or not to act on that recommendation. Or you can bypass human input altogether and automate your decision-making.
No results found.
GUROBI NEWSLETTER
Latest news and releases